Does your enterprise lack the infrastructure necessary to support disruptive technologies?
Our network can handle this, right? Right?
This “question” has enterprise network managers squirming in their seats in conference rooms around the world.
Some have a ready answer. Others are not so sure. The majority struggle to come up with a simple explanation for an incredibly complex situation that has multiple moving parts. It’s especially difficult when the question comes from a non-technical type of person.
Why the sudden interest in the network?
Nearly all industry sectors are deploying “disruptive” technologies to compete for increasingly demanding customers, while maintaining a tight control on expenses. These “disruptive” technologies include Artificial Intelligence, Cognitive Systems, Robotic Process Automation, blockchain, Quantum Computing, and billions of devices connected through the Internet of Things.
Artificial intelligence (AI) and machine learning (ML) are foundations that enables the other technologies. The combination of AI and ML with the others sparks the question about network readiness.
- Healthcare systems combine machine learning, genomic sequencing, 3D imaging and robot-assisted surgery to improve patient outcome, reduce hospital readmission and decrease cost.
- Smart Cities merge sensors, video cameras and other devices with deep neural networks, unmanned vehicles, and chatbots to deliver quality government services while maintaining fiscal responsibility.
- Financial services firms mix predictive regression algorithms, robotic process automation and block chain to provide customized “open-banking” services, streamline operations and enhance data security.
The reason for the renewed focus on connectivity stems from the sheer volumes of data generated by these combinations. AI/ML-powered applications require constant inputs of data from a wide variety of sources. For instance, a basic machine learning classification application like medical image diagnosis or vehicle identification can involve millions of high-resolution images generated from numerous video and photo devices and data bases. Those that need to be analyzed in real-time add an additional degree of complexity.
A basic machine learning classification application like medical image diagnosis or vehicle identification can involve millions of high-resolution images generated from numerous video and photo devices and data bases.
Don’t make the wrong assumption
While the cloud is emerging as a major resource for AI and ML based workloads, many enterprises assume that they can rely on their on-premises storage environment and legacy network infrastructure for these projects. Network managers that are consulted early enough in the process can evolve in time to support the new demands.
Unfortunately, these appear to be in the minority. A recent McKinsey & Company study found that most companies lack the digital technology infrastructure required to support AI applications.
This coincides with conversations I’ve had with network professionals and during my speaking sessions at diverse industry conferences. They agree that to provide the high-efficiency network resources at scale required to support these technology combinations, networks will need to be evolve. Scalability, openness, security, intelligent automation and “bandwidth on-demand” need to be key components of any network architecture.
Evolutions in network technology can help
AI and ML are being combined with programmable network equipment, software-based control and virtualization to support the connectivity requirements of these disruptive technologies.
Armed with these capabilities, when confronted with the “question”, network managers will no longer feel “on the hot seat”.
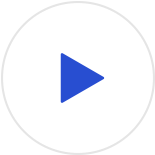
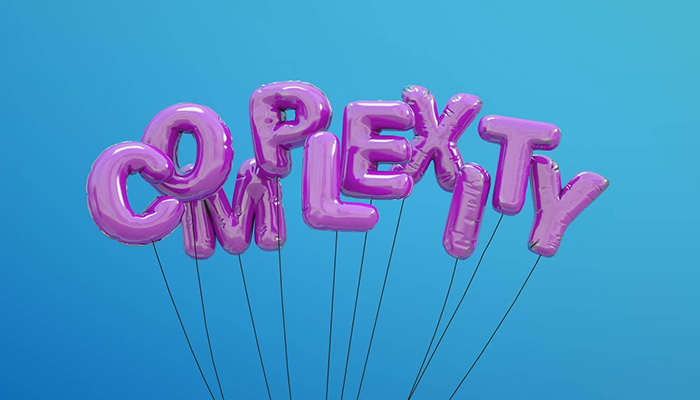
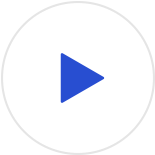